Generative AI Use Cases in Data Management
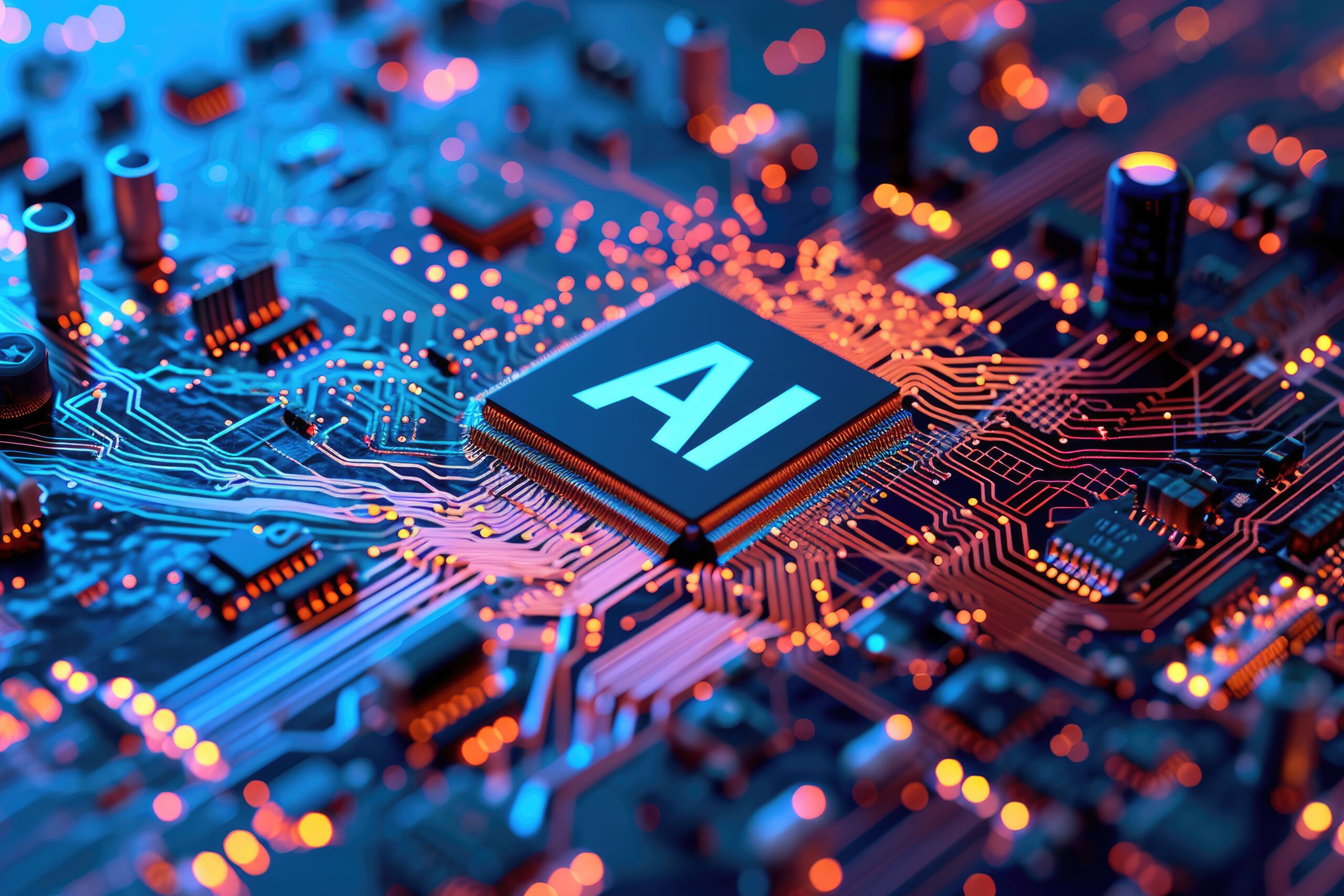
In the digital era, organizations generate and process vast amounts of data across multiple systems, making efficient data management a critical necessity. Traditional data management strategies rely on rule-based systems, static metadata repositories, and manual interventions. However, with the advent of Generative AI, enterprises can revolutionize their data management practices by automating data governance, improving data quality, and optimizing data storage and retrieval processes.
The Use of Generative AI for Data Management
Generative AI utilizes deep models and massive-scale data processing for optimizing several processes in data management, such as:
✅ Data Discovery & Cataloging – To automate finding, categorization, and data storage assets in diverse locations.
✅ Data Quality & Anomaly Detection – Identifying errors, empty fields, and anomalies beforehand using machine learning-based profiling of data.
✅ Automated Metadata Management – Real-time generation of metadata to deliver enriched context to structured and unstructured information.
✅ Data Lineage & Compliance – Data transformation tracking and documentation to enable regulatory compliance like GDPR, HIPAA, and CCPA.
✅ Intelligent Data Integration – Enhancing data interoperability by automatically mapping relationships between disparate datasets.
Generative AI Use Cases in Data Management
1. AI-Powered Data Lineage and Governance
Traditional Challenge:
Organizations grapple with dispersed data lineage documentation, which makes it hard to identify data sources, transformations, and consumption.
Generative AI Solution:
Generative AI models can map data lineage automatically by:
- Consuming metadata from platforms such as Collibra, Informatica, and Alation.
- Utilizing natural language processing (NLP) to derive lineage information from business glossaries and system logs.
- Enabling real-time lineage visualization to meet data governance regulations.
2. Data Quality Improvement with AI-Driven Anomaly Detection
Traditional Challenge:
Data inconsistencies and anomalies tend to go undetected, causing reporting and analytics errors.
Generative AI Solution:
AI can constantly monitor datasets and:
- Detect and mark outliers with sophisticated anomaly detection models.
- Recommend corrections and transformations based on past data trends.
- Lower manual data cleansing tasks, enhancing operational productivity.
3. Smart Data Classification & Tagging
Traditional Challenge:
Data classification is time-consuming and error-prone when done manually.
Generative AI Solution:
AI models can:
- Automatically label sensitive information (PII, financial info, healthcare records) for compliance.
- Enhance metadata labeling to enhance search and retrieval.
- Enable security policy enforcement by enforcing role-based access controls dynamically.
4. AI-Optimized Data Storage and Cost Savings
Traditional Challenge:
Companies tend to overprovision storage, resulting in higher costs and inefficiencies.
Generative AI Solution:
AI-powered data storage optimization assists with:
- Identifying duplicate or unused datasets and recommending deletion or archiving.
- Predicting storage capacity needs based on historical usage trends.
- Automating data lifecycle management to balance performance and cost.
5. AI-Based Data Integration & Interoperability
Traditional Challenges:
Enterprises struggle with integrating disparate data sources due to inconsistencies in formats and schemas.
Generative AI Solution:
By using machine learning-based data mapping, AI can:
- Automate schema mappings to integrate data with ease.
- Identify and rectify data discrepancies between systems.
- Facilitate real-time synchronization of data in multi-cloud setups.
The Future of AI for Data Management
By leveraging Generative AI, organizations can achieve:
✅ Improved Data Governance – Automating compliance tracking and policy enforcement.
✅ Increased Data Quality – Catching errors and inconsistencies in real-time.
✅ Better Costs – Minimizing redundant storage and processing overhead.
✅ Better Decision-Making – Allowing timely and accurate access to data.
✅ Scalability & Automation – Minimizing manual intervention in data management tasks.
Next Steps for Enterprises
1️⃣ Implement AI-based data lineage and governance frameworks.
2️⃣ Incorporate Generative AI models into metadata management processes.
3️⃣ Apply AI to identify anomalies in real time and perform quality checks.
4️⃣ Roll out AI-based recommendations for cost optimization and storage.
5️⃣ Use AI for smooth data integration between business applications.
Through Generative AI-enabled data management, businesses can construct a more dynamic, intelligent, and compliant data environment—providing insurance for future-ready operations in the ever-growing era of data intensity.
One thought on "Generative AI Use Cases in Data Management"
Great article! Very informative on how generative AI is transforming data management. Thanks for sharing!