Entity Extraction using Gen AI
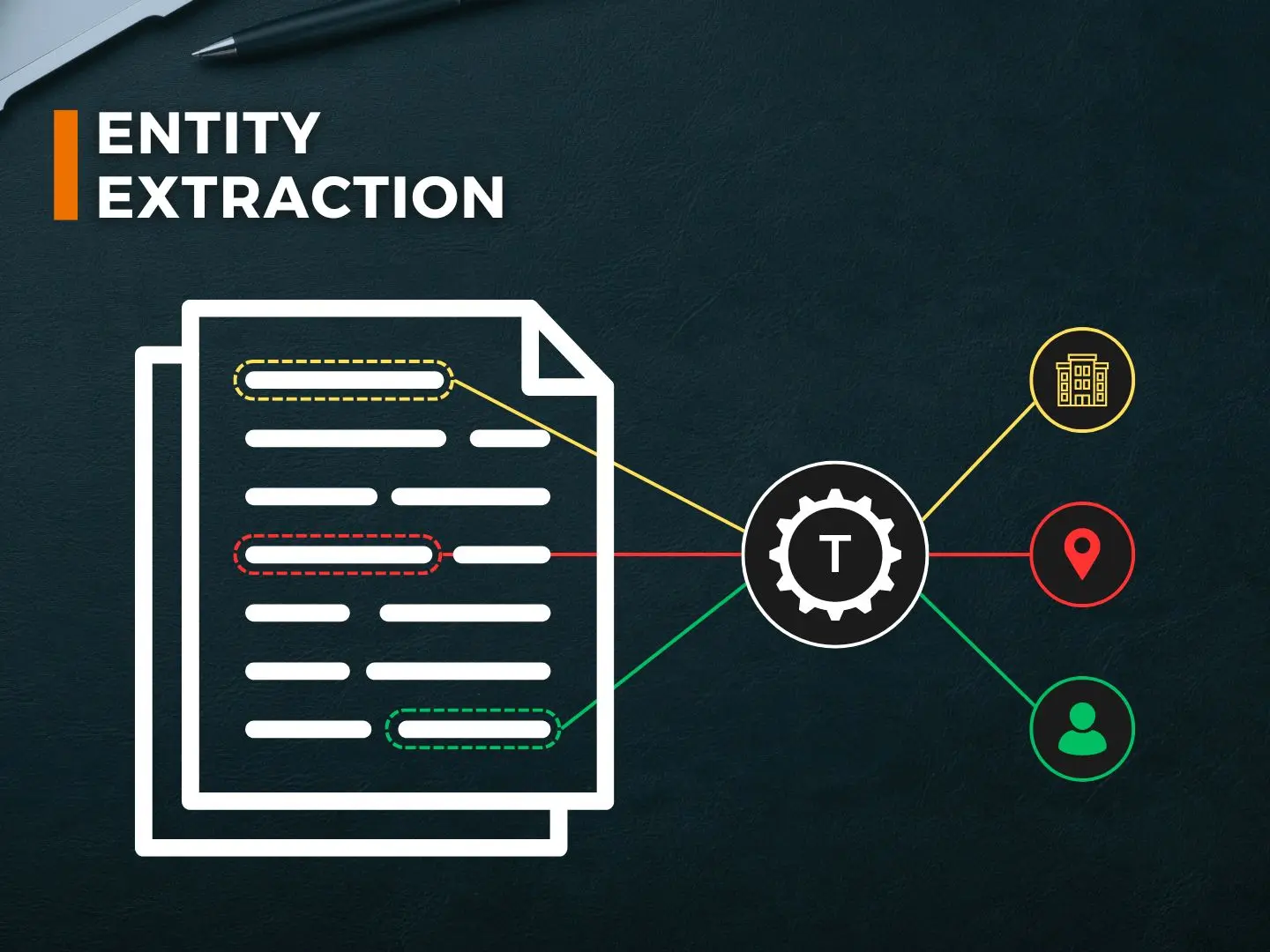
In today’s data-driven business, deriving useful information from unstructured data is a growing challenge. Entity Extraction based on Generative AI allows organizations to extract and categorize important entities like names, locations, organizations, and concepts from large data sets. Conventional entity extraction techniques often have difficulties with context, ambiguity, and changing data structures.
However, through AI-based metadata management, companies can automate entity extraction, increase accuracy, and better optimize downstream analytics and decision-making.
The Role of Metadata in Entity Extraction
Metadata plays a crucial role in structuring and managing the entity extraction process. It supplies the contextual backbone for proper entity identification, association, and categorization. The significant metadata-driven advantages in entity extraction include:
- Better Data Organization – Metadata groups and organizes extracted entities to facilitate retrieval and analysis.
- Contextual Relevance – AI systems use metadata to resolve entities with identical names but distinct meanings.
- Regulatory Compliance – Metadata-based entity monitoring enables organizations to comply with GDPR, HIPAA, and regulatory requirements.
- Semantic Enrichment – Metadata enriches extracted entities by connecting them with similar terms, synonyms, and ontologies.
Techniques in Entity Extraction
Entity extraction utilizes sophisticated methods to recognize and categorize entities with high accuracy. Some of the most popular methods are:
- Rule-Based Methods – Applying pre-defined patterns and regular expressions to identify certain entities.
- Statistical and Machine Learning Models – Utilizing NLP models like CRF, LSTMs, and Transformer-based models for entity recognition.
- Deep Learning-Based Extraction – Employing Generative AI models such as GPT and BERT to deduce entity relationships and classify them contextually.
- Hybrid Models – Integrating rule-based and ML methods to enhance accuracy and flexibility.
Integration with Generative AI Models
Generative AI models have revolutionized entity extraction by making the following possible:
1️⃣ Better Context Comprehension – AI models analyze large corpora to comprehend the subtle meanings of entities.
2️⃣ Self-Learning Entity Recognition – AI learns to recognize new entity types through continuous learning without being explicitly programmed.
3️⃣ Automated Disambiguation – Generative AI distinguishes ambiguous entities based on contextual information.
4️⃣ Dynamic Entity Expansion – Model-based AI proposes related entities, synonyms, and variations for complete extraction.
Use of Ontologies and Knowledge Graphs
Ontologies and knowledge graphs improve entity extraction by giving structured relationships between entities, improving accuracy and contextual understanding.
✅ Ontology-Driven Classification – Predefined ontologies classify entities into useful taxonomies.
✅ Knowledge Graph Augmentation – AI models utilize knowledge graphs (e.g., Wikidata, Google Knowledge Graph) to connect extracted entities with validated data sources.
✅ Enhanced Query Understanding – Contextual entity linking makes search engines and chatbots more relevant.
Metadata Annotation and Tagging
Metadata annotation and tagging are crucial in organizing extracted entities for enhanced usability and searchability.
✅ AI-Generated Annotations – Generative AI models automatically provide meaningful metadata to extracted entities.
✅ Semantic Tagging – AI-based metadata tagging enhances the classification and retrieval of unstructured data.
✅ Real-Time Metadata Enrichment – Ongoing learning allows AI to enrich entity metadata in real time.
Entity Extraction Challenges
Despite its benefits, entity extraction presents the following challenges:
❌ Data Ambiguity – Picking out the correct entities from ambiguous, unstructured text is still challenging.
❌ Scalability Issues – Entity extraction on a large scale demands extensive computational power.
❌ Regulatory Constraints – Dealing with entity metadata while complying with data privacy laws.
❌ Multilingual Challenges – Entity extraction from multilingual content demands high-powered NLP capabilities.
Automation in Metadata Management
Automation becomes essential in automating metadata to optimize entity extraction. The principal advantages of metadata automation using AI are:
- Real-Time Metadata Updates – AI continuously updates entity metadata as new information is fed.
- Self-Learning Systems – AI repeatedly perfects metadata tagging according to changing data patterns.
- Scalable Entity Processing – Pipelines with automation process large amounts of unstructured data seamlessly.
- Regulatory Compliance Assurance – AI makes metadata compliant with industry standards and privacy regulations.
The Future of AI-Powered Entity Extraction
By combining Generative AI with entity extraction, organizations can accomplish the following:
- Automated, high-accuracy entity recognition
- Better metadata-driven data governance
- Scalable entity extraction across verticals
- Self-optimizing AI pipelines for metadata tagging
- Seamless integration with knowledge graphs and ontologies
By leveraging Generative AI in Entity Extraction, enterprises can transform their data ecosystems, ensuring accurate, efficient, and scalable metadata management for future-proofed business operations.